What Is The Main Reason For Bias In The Ai Systems
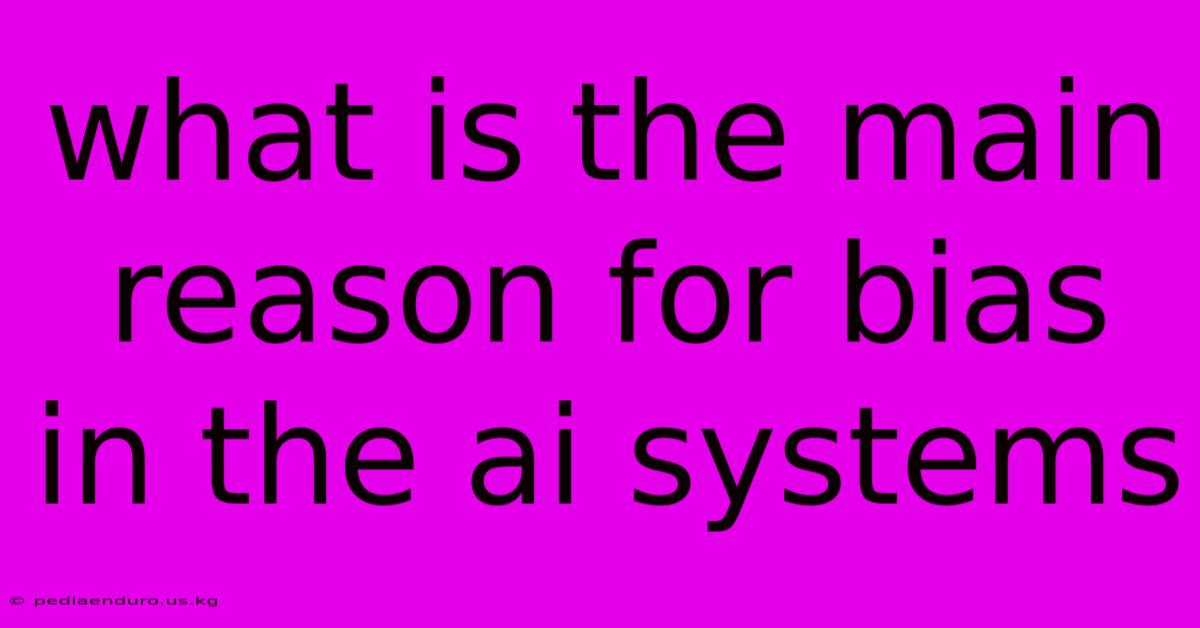
Discover more detailed and exciting information on our website. Click the link below to start your adventure: Visit Best Website mr.meltwatermedia.ca. Don't miss out!
Table of Contents
The Main Reason for Bias in AI Systems: A Deep Dive into Data's Shadow
The rapid advancement of Artificial Intelligence (AI) has ushered in an era of unprecedented technological possibilities. However, a significant shadow looms over this progress: bias. AI systems, despite their seemingly objective nature, often perpetuate and even amplify existing societal biases, leading to unfair or discriminatory outcomes. While various factors contribute to this problem, the primary reason for bias in AI systems boils down to one crucial element: biased data.
This article will delve into the multifaceted nature of bias in AI, exploring the inherent biases within datasets, the mechanisms through which these biases manifest in AI models, and the critical steps required to mitigate this pervasive issue. We will examine the role of human involvement, the complexities of algorithmic fairness, and the ethical implications of deploying biased AI systems.
The Data Problem: A Foundation of Bias
AI systems, particularly those employing machine learning techniques, learn from data. They identify patterns, relationships, and trends within vast datasets to make predictions and decisions. The fundamental problem is that much of the data used to train these systems reflects existing societal biases. These biases aren't necessarily intentional; they are often subtle and embedded within the data collection, representation, and annotation processes.
Consider these examples:
-
Facial Recognition: Many facial recognition systems exhibit higher error rates for individuals with darker skin tones. This isn't because the algorithms are inherently racist, but because they were trained on datasets that heavily overrepresented lighter skin tones, resulting in a model that performs less accurately on underrepresented groups.
-
Loan Applications: AI systems used to assess loan applications might inadvertently discriminate against certain demographic groups if the training data reflects historical lending practices that were themselves biased. For example, if past data shows a higher default rate for a particular group, the AI might unfairly deny loans to individuals from that group, even if their current financial situation warrants approval.
-
Hiring Processes: AI tools used to screen resumes might perpetuate gender bias if the training data reflects historical hiring practices where certain genders were favored over others. The AI might learn to associate certain names or keywords with specific genders and then unfairly prioritize or reject candidates based on these associations.
These examples highlight how historical and societal biases, whether conscious or unconscious, are encoded in the data. This biased data acts as the foundation upon which AI systems are built, leading to biased outcomes.
Mechanisms of Bias Amplification:
The biases embedded in data aren't simply passively reflected in AI systems; they are often amplified through various mechanisms:
-
Data Representation: The way data is collected and represented can significantly influence the outcome. For example, if a dataset representing customer service interactions omits crucial context or uses subjective labels, the AI might learn to associate certain customer demographics with negative behaviors, even if those behaviors are the result of systemic issues or unequal access to resources.
-
Algorithmic Bias: Even with unbiased data, the algorithms themselves can introduce bias. The choice of algorithm, the way features are selected and weighted, and the optimization criteria can all influence the fairness of the AI system. Some algorithms are inherently more susceptible to amplifying existing biases in the data.
-
Feedback Loops: Once deployed, AI systems often interact with the real world, generating new data that can further reinforce existing biases. For example, if a biased hiring system consistently favors candidates from a particular background, it will create a workforce that further reinforces the bias in future datasets used to train the system. This creates a self-perpetuating cycle of bias.
Beyond Data: The Human Element
While biased data is the primary culprit, it’s crucial to acknowledge the role of human factors. Bias is not only present in the data; it is also embedded in the choices made by the developers, designers, and users of AI systems.
-
Developer Bias: The developers themselves might have unconscious biases that influence their choices regarding data collection, algorithm selection, and model evaluation. This can inadvertently lead to the creation of systems that perpetuate these biases.
-
Data Annotation Bias: The process of annotating data (labeling and categorizing information) is often done by humans, and human annotators can introduce their own biases into the data. Inconsistent or biased annotation can significantly impact the performance and fairness of the AI system.
Mitigating Bias: A Multifaceted Approach
Addressing bias in AI requires a multi-pronged approach that tackles the problem at its source: the data. Here are some key strategies:
-
Data Augmentation: Increasing the representation of underrepresented groups in the datasets can help reduce bias. This can involve actively collecting more data from marginalized groups or using techniques to synthetically generate data that represents these groups.
-
Data Preprocessing: Techniques such as re-weighting, re-sampling, and adversarial debiasing can help mitigate bias in existing datasets. These methods aim to balance the representation of different groups and reduce the influence of biased features.
-
Algorithm Selection: Choosing algorithms that are less susceptible to bias and incorporating fairness constraints into the optimization process can improve the fairness of the AI system.
-
Transparency and Explainability: Making AI systems more transparent and explainable can help identify and address sources of bias. This allows developers and users to understand how the system makes decisions and identify potential points of bias.
-
Human Oversight: Incorporating human oversight into the AI development process can help identify and correct biases before they manifest in deployed systems. This can involve having human reviewers assess the fairness and accuracy of the AI's decisions.
-
Continuous Monitoring and Evaluation: Regularly monitoring and evaluating the performance of AI systems for fairness and accuracy is crucial to identifying and addressing emerging biases.
Ethical Considerations:
The presence of bias in AI systems raises significant ethical concerns. Biased AI systems can lead to discriminatory outcomes in various domains, exacerbating existing inequalities and causing harm to individuals and communities. It is crucial that developers, policymakers, and users of AI systems prioritize fairness, accountability, and transparency to ensure that AI benefits everyone and does not exacerbate societal divides.
Conclusion:
The main reason for bias in AI systems is the presence of bias in the data used to train them. This bias is often subtle and embedded within the data collection, representation, and annotation processes, but its effects are profound and far-reaching. Mitigating this bias requires a comprehensive approach that addresses both the data and the human factors involved in AI development and deployment. By actively working towards more equitable and representative datasets, adopting fair algorithms, and promoting transparency and accountability, we can strive to create AI systems that serve all members of society justly and fairly. The journey towards unbiased AI is a continuous process of learning, refinement, and ethical reflection.
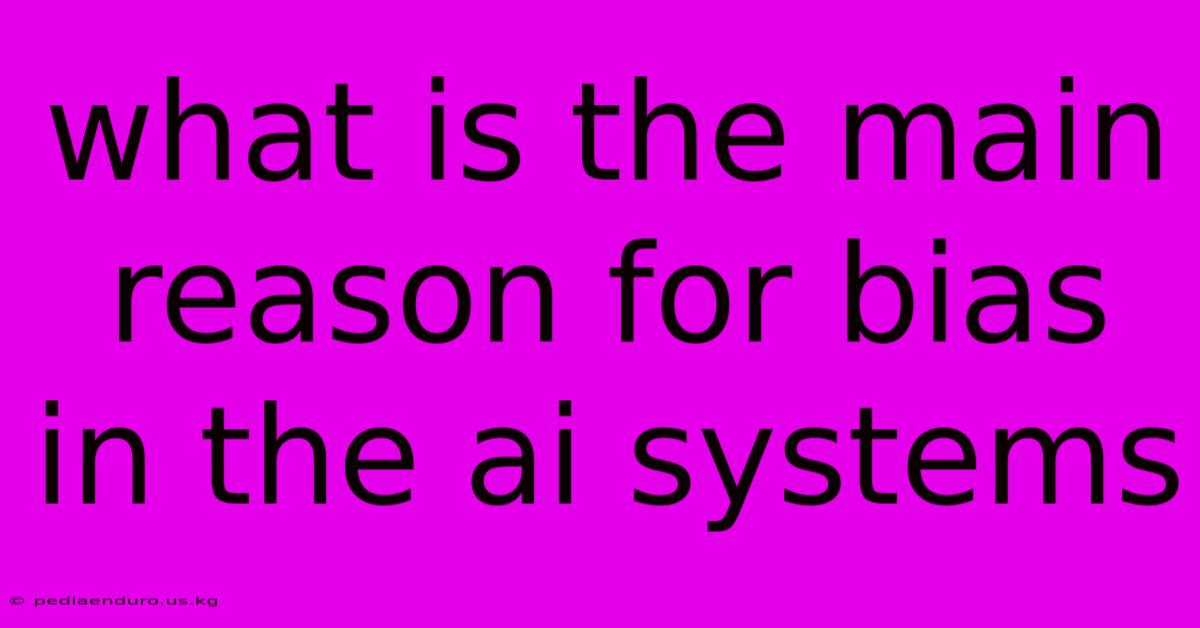
Thank you for visiting our website wich cover about What Is The Main Reason For Bias In The Ai Systems. We hope the information provided has been useful to you. Feel free to contact us if you have any questions or need further assistance. See you next time and dont miss to bookmark.
Also read the following articles
Article Title | Date |
---|---|
Bing Ai Image Creator 3d Love | Feb 03, 2025 |
Bianca Censori Sa Grammys 2025 | Feb 03, 2025 |
Ac Milan Vs Inter Laga Sengit 1 1 | Feb 03, 2025 |
Bing Ai Chatbot Kevin Roose | Feb 03, 2025 |
Bing Ai Create Account | Feb 03, 2025 |