How Does Ai Art Work
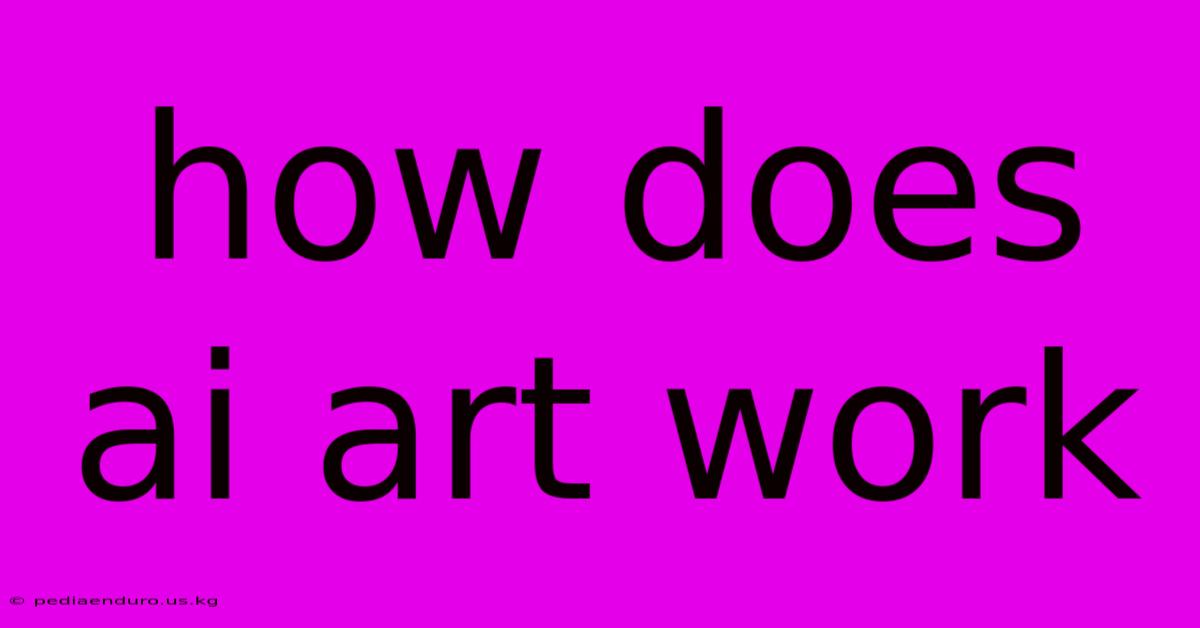
Discover more detailed and exciting information on our website. Click the link below to start your adventure: Visit Best Website mr.meltwatermedia.ca. Don't miss out!
Table of Contents
How Does AI Art Work? Unveiling the Magic Behind the Algorithms
The rise of AI art has captivated the world, sparking both awe and apprehension. Images generated by algorithms seem to possess a creative spark, raising questions about the nature of art and the future of creative professions. But how does this seemingly magical process actually work? The answer lies in a complex interplay of deep learning, massive datasets, and sophisticated algorithms. This article delves into the fascinating mechanics behind AI art generation, exploring the different approaches and the underlying technologies that make it possible.
Catatan Editor: Artikel ini diterbitkan hari ini untuk memberikan wawasan terbaru tentang bagaimana seni AI bekerja.
The Importance of Understanding AI Art
In a world increasingly driven by technology, understanding AI art is not merely a matter of intellectual curiosity. It's crucial for artists, designers, and anyone interested in the creative landscape. AI art is not just a fleeting trend; it's a powerful tool with the potential to revolutionize creative processes, fostering collaboration between humans and machines, and opening up new avenues for artistic expression. Understanding its underlying mechanisms allows us to appreciate its capabilities, limitations, and its ethical implications.
A Deep Dive into the Process: From Data to Image
AI art generation primarily relies on a type of deep learning model called a Generative Adversarial Network (GAN). A GAN consists of two neural networks, locked in a constant game of "cat and mouse":
-
The Generator: This network tries to create realistic images based on the input it receives. It's like a forger, constantly refining its techniques to produce convincing counterfeits.
-
The Discriminator: This network acts as the art critic, evaluating the images generated by the generator. Its job is to distinguish between real images from a training dataset and the fakes created by the generator.
The two networks are trained simultaneously. The generator learns by trying to fool the discriminator, while the discriminator learns to better identify the generator's creations. This adversarial process leads to a continuous improvement in both networks, with the generator becoming increasingly adept at producing realistic and creative images.
The Role of Data: Feeding the Beast
The success of a GAN hinges on the quality and quantity of its training data. These models are trained on massive datasets containing millions of images. The more diverse and representative the dataset, the more versatile and creative the resulting AI art generator will be. These datasets can include images scraped from the internet, curated collections of artworks, or even specialized datasets focusing on specific styles or subjects.
The process of preparing this data is critical. Images need to be properly formatted, cleaned (removing noise or irrelevant information), and labeled if necessary. This data preparation step is often labor-intensive and requires specialized expertise.
Beyond GANs: Other AI Art Techniques
While GANs are prevalent, other techniques contribute significantly to the AI art landscape:
-
Variational Autoencoders (VAEs): These models excel at compressing and reconstructing images, allowing for the generation of novel images by manipulating the latent space representation of the data. They are particularly useful for generating images with specific characteristics or manipulating existing images.
-
Diffusion Models: These models work by adding noise to an image until it's completely random, then learning to reverse this process, generating new images from noise. They often produce incredibly detailed and high-quality results.
-
Transformer Networks: Inspired by natural language processing, these models are increasingly used in AI art to generate images from textual descriptions (text-to-image generation). They can understand complex prompts and generate images that closely align with the given descriptions.
Decoding the Process: A Step-by-Step Breakdown (using GANs as an example)
-
Data Input: The GAN is fed a massive dataset of images.
-
Generator Creation: The generator creates a random image based on the learned patterns from the data.
-
Discriminator Evaluation: The discriminator assesses the generated image, determining whether it's real or fake.
-
Feedback Loop: The discriminator's feedback is used to update both the generator and the discriminator's weights. The generator learns from its mistakes, trying to produce more realistic images, while the discriminator becomes better at identifying fakes.
-
Iteration: This process repeats thousands, even millions, of times, leading to the generator's ability to generate increasingly realistic and creative images.
-
Output: The final output is an image generated by the generator, often indistinguishable from images created by humans.
The Human Element: Guiding the Algorithm
Although AI art generation is driven by algorithms, human intervention remains crucial. Artists often use various techniques to guide the creative process:
-
Prompt Engineering: Carefully crafting textual prompts is vital for generating desired results in text-to-image models. The nuances of language significantly influence the generated image.
-
Parameter Tuning: Adjusting the parameters of the model (e.g., learning rate, number of iterations) can fine-tune the output, affecting style, realism, and other aspects of the generated image.
-
Post-Processing: Generated images often undergo post-processing by human artists to refine details, add finishing touches, or integrate them into larger artworks.
Frequently Asked Questions about AI Art
-
What is AI art and why is it important? AI art is art created with the assistance of artificial intelligence algorithms. It's important because it pushes the boundaries of artistic expression, explores new creative methods, and raises fascinating questions about the nature of creativity and art itself.
-
How does AI art work? AI art primarily uses generative models, such as GANs, VAEs, or diffusion models, trained on vast datasets of images. These models learn patterns and styles from the data and generate new images based on these learnings.
-
What are the main benefits of AI art? Benefits include increased accessibility to art creation tools, exploration of novel artistic styles, and the ability to generate personalized art.
-
What are the challenges associated with AI art? Challenges include ethical concerns regarding copyright and ownership, the potential for bias in training data, and the impact on human artists.
-
How can I start creating AI art? There are numerous online platforms and tools that allow you to generate AI art, often with minimal technical expertise required.
Tips for Working with AI Art Tools
- Experiment with different platforms and models: Each tool has its strengths and weaknesses; explore different options to find what suits your style.
- Refine your prompts carefully: The quality of your prompts directly impacts the quality of the generated art.
- Iterate and refine: Don't expect perfect results on the first try. Experiment and refine your approach to achieve desired outcomes.
- Learn about the underlying technologies: A deeper understanding of the technology will help you better utilize the tools and achieve more creative results.
- Embrace collaboration: Consider AI as a collaborative partner rather than a replacement for human creativity.
Summary of Key Points about AI Art
AI art represents a significant leap forward in creative technology, blending computational power with human imagination. While the process involves complex algorithms and massive datasets, the human element remains crucial, guiding the process and shaping the final outcome. Understanding the mechanics of AI art generation allows us to appreciate its potential and address the ethical implications that come with this exciting new frontier. The future of art likely involves a harmonious collaboration between human creativity and the power of artificial intelligence, pushing the boundaries of artistic expression in ways previously unimaginable. The journey has just begun.
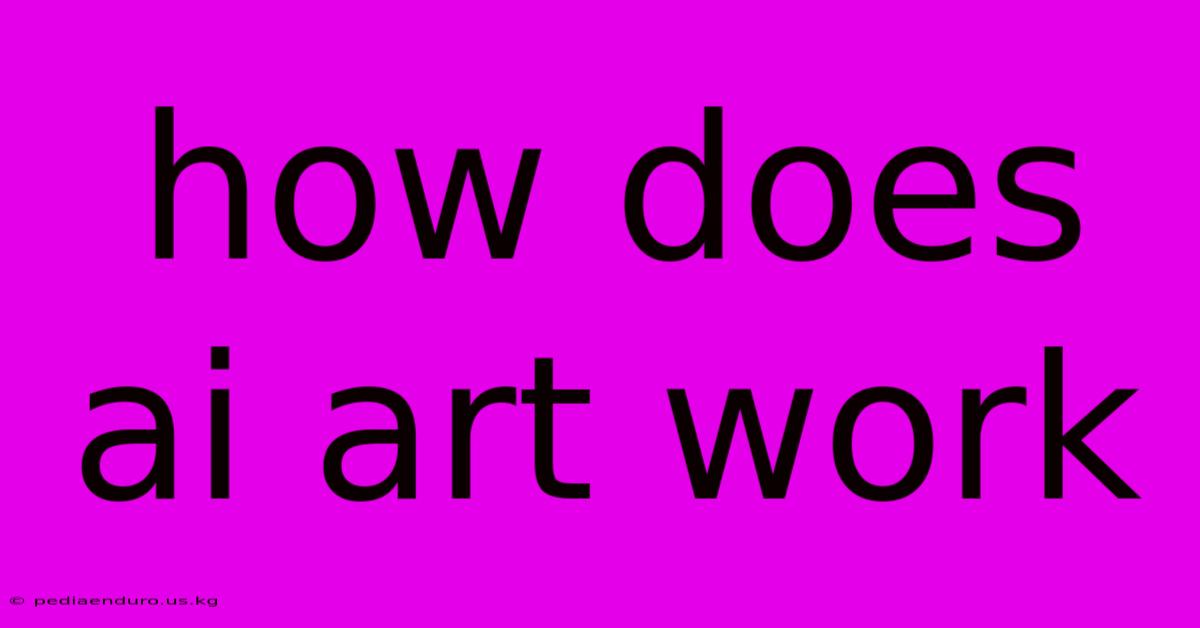
Thank you for visiting our website wich cover about How Does Ai Art Work. We hope the information provided has been useful to you. Feel free to contact us if you have any questions or need further assistance. See you next time and dont miss to bookmark.
Also read the following articles
Article Title | Date |
---|---|
Is Bing Ai App Good | Feb 03, 2025 |
Bing Ai Creator 3d | Feb 03, 2025 |
Pagpanaw Ni Barbie Hsu Katotohanan | Feb 03, 2025 |
Bing Ai Office 365 | Feb 03, 2025 |
Bing Dan Bong | Feb 03, 2025 |