How Do Ai Startups Make Money
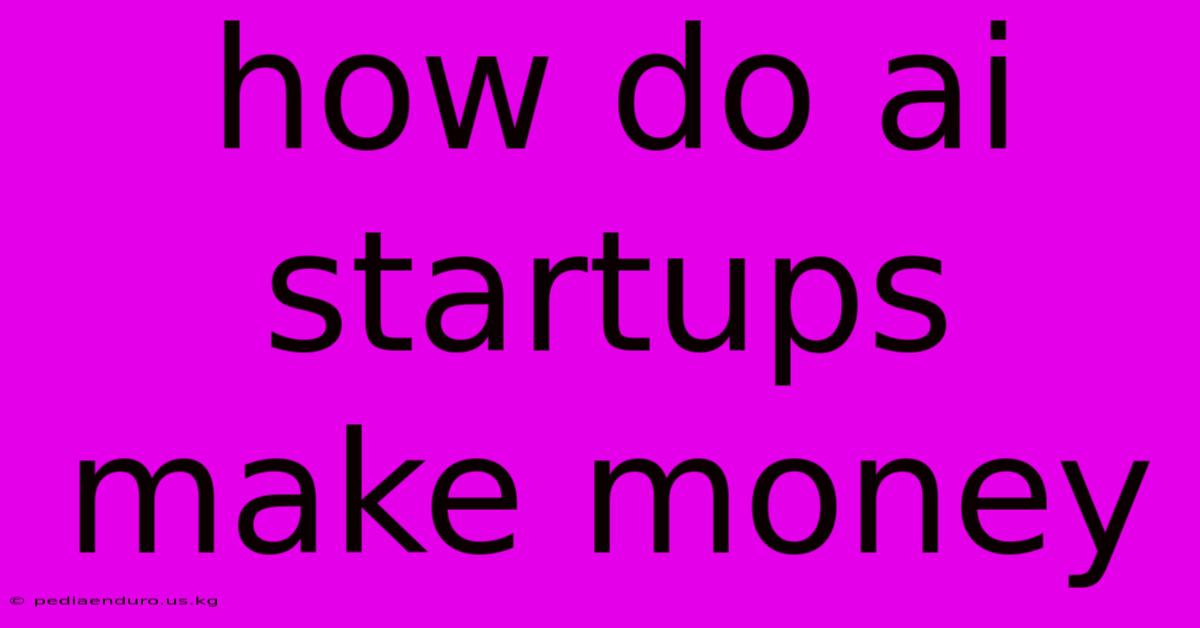
Discover more detailed and exciting information on our website. Click the link below to start your adventure: Visit Best Website mr.meltwatermedia.ca. Don't miss out!
Table of Contents
How Do AI Startups Make Money? A Deep Dive into Revenue Models
The artificial intelligence (AI) industry is booming, attracting significant investment and fostering a surge in innovative startups. But amidst the excitement and technological breakthroughs, a crucial question remains: how do these AI startups actually make money? The answer isn't simple, as revenue models vary significantly depending on the specific AI technology, target market, and stage of development. This article delves deep into the diverse ways AI startups generate revenue, exploring their strategies, challenges, and future prospects.
Hook: What's the secret sauce behind the financial success of AI startups, and how are they navigating the complex landscape of monetization?
Note from the Editor: This article was published today to provide the latest insights into the diverse revenue models employed by AI startups.
Relevance: Understanding the business models of AI startups is crucial for investors, entrepreneurs, and anyone interested in the future of this rapidly evolving field. The insights provided here offer a valuable framework for analyzing the viability and potential of AI ventures.
In-depth Analysis: This article synthesizes information from various sources, including industry reports, case studies of successful AI startups, and interviews with experts in the field. It aims to provide a comprehensive overview of the prevalent revenue models, analyzing their strengths and weaknesses.
Key Takeaways:
Point | Explanation |
---|---|
Software Licensing | Selling access to AI software or algorithms. |
API Access | Charging for access to AI APIs (Application Programming Interfaces). |
Data Services | Providing data annotation, labeling, or cleaning services. |
Consulting Services | Offering expertise and custom AI solutions for businesses. |
SaaS (Software as a Service) | Delivering AI-powered software applications through subscription models. |
Hardware Sales | Selling specialized hardware required for AI processing. |
Product Integration | Embedding AI capabilities into existing products or services. |
Platform Fees | Charging fees for access to or usage of an AI platform. |
M&A (Mergers & Acquisitions) | Selling the company to a larger entity. |
Transition: Now that we’ve established the various avenues for revenue, let's explore each in detail.
I. Software Licensing: This traditional model involves selling the right to use AI software, often with different licensing tiers based on features or usage limits. This approach is suitable for startups with strong intellectual property (IP) in specialized AI algorithms, such as those for medical image analysis or financial modeling. The challenge lies in securing a broad enough customer base to achieve profitability and protecting the IP from unauthorized use.
II. API Access: Many AI startups provide access to their algorithms and models through APIs, allowing other developers and businesses to integrate AI capabilities into their own applications. This model typically uses a pay-per-use or subscription-based pricing structure. The advantage is scalability – a single API can serve a large number of customers. However, competition can be fierce, requiring startups to offer superior performance, reliability, and ease of integration.
III. Data Services: High-quality, labeled data is essential for training effective AI models. AI startups often capitalize on this by offering data annotation, cleaning, or labeling services. This is a particularly lucrative segment, especially with the increasing demand for data in machine learning applications. The challenge lies in securing reliable data sources and efficiently managing the annotation process.
IV. Consulting Services: Many AI startups provide consulting services, helping businesses implement AI solutions tailored to their specific needs. This could involve developing custom AI models, integrating existing AI technologies, or providing strategic guidance on AI adoption. While this model offers high profit margins, it's often less scalable than others. The success hinges on the expertise and reputation of the consultants.
V. SaaS (Software as a Service): This increasingly prevalent model delivers AI-powered applications through a subscription. This can range from simple AI tools for marketing automation to complex enterprise resource planning (ERP) systems enhanced with AI. The advantages include recurring revenue streams and ease of deployment. Competition is intense, and success demands continuous product improvement and strong customer retention.
VI. Hardware Sales: Some AI startups focus on developing specialized hardware for AI processing, such as GPUs (Graphics Processing Units) or AI accelerators. This model is capital-intensive but can be highly profitable if the hardware achieves widespread adoption. However, the market is dominated by large players, making it challenging for startups to compete.
VII. Product Integration: This involves embedding AI capabilities into existing products or services to enhance their functionality. For example, AI-powered features can be added to cameras, smartphones, or enterprise software. This approach offers significant market reach but requires strong partnerships with established companies.
VIII. Platform Fees: Some AI startups build platforms that allow developers to build and deploy their AI applications. They then charge fees for access to the platform's resources and services. This model has significant potential for scalability but requires creating a valuable and attractive platform that draws a significant developer community.
IX. Mergers & Acquisitions (M&A): While not a revenue model per se, many successful AI startups eventually become acquisition targets for larger tech companies. This provides a significant exit strategy for investors and founders, although it’s not a guaranteed outcome.
FAQ about AI Startup Revenue Models:
What are AI startup revenue models and why are they important?
AI startup revenue models describe how these companies generate income. Understanding these models is crucial for investors, assessing the financial viability of a venture, and for AI startups themselves to strategize for growth and sustainability.
How do different AI startup revenue models work?
The models vary significantly, from licensing software to providing data services, SaaS subscriptions, and selling hardware. Each model has unique challenges and advantages regarding scalability, profit margins, and market competition.
What are the main benefits of different AI startup revenue models?
Benefits range from high profit margins (consulting), recurring revenue streams (SaaS), and large-scale potential (API access) to less reliance on sales cycles (SaaS).
What are the common challenges faced by AI startups regarding monetization?
Challenges include high initial investment costs, intense competition, the need for specialized expertise, securing enough data for training models, and protecting IP.
How can an AI startup choose the right revenue model?
The choice depends on factors such as the specific AI technology, target market, competitive landscape, and the startup's resources and capabilities.
Summary of the FAQ: Choosing the appropriate revenue model is vital for AI startup success, requiring a deep understanding of the market and the specific capabilities of the technology being offered.
Tips for AI Startups on Monetization:
- Identify a clear value proposition: Clearly articulate how your AI solution addresses a specific problem for your target customers.
- Prioritize customer acquisition: Develop a robust marketing and sales strategy to reach your target audience.
- Develop a strong pricing strategy: Consider factors such as pricing tiers, payment options, and perceived value.
- Focus on customer retention: Building strong customer relationships is crucial for sustainable growth.
- Monitor key metrics: Closely track key performance indicators (KPIs) such as customer acquisition cost, churn rate, and average revenue per user.
Summary of the Article: AI startups employ a variety of revenue models, from traditional software licensing to cloud-based SaaS solutions and data services. Success requires a clear understanding of the target market, a strong value proposition, and a well-defined go-to-market strategy. The future of AI monetization will likely involve increasingly sophisticated hybrid models and a focus on delivering real-world value to businesses and consumers.
Concluding Message: The AI landscape is constantly evolving, and the strategies for monetization will continue to adapt. Startups that can innovate not just in technology but also in their business models will be best positioned for long-term success in this dynamic industry.
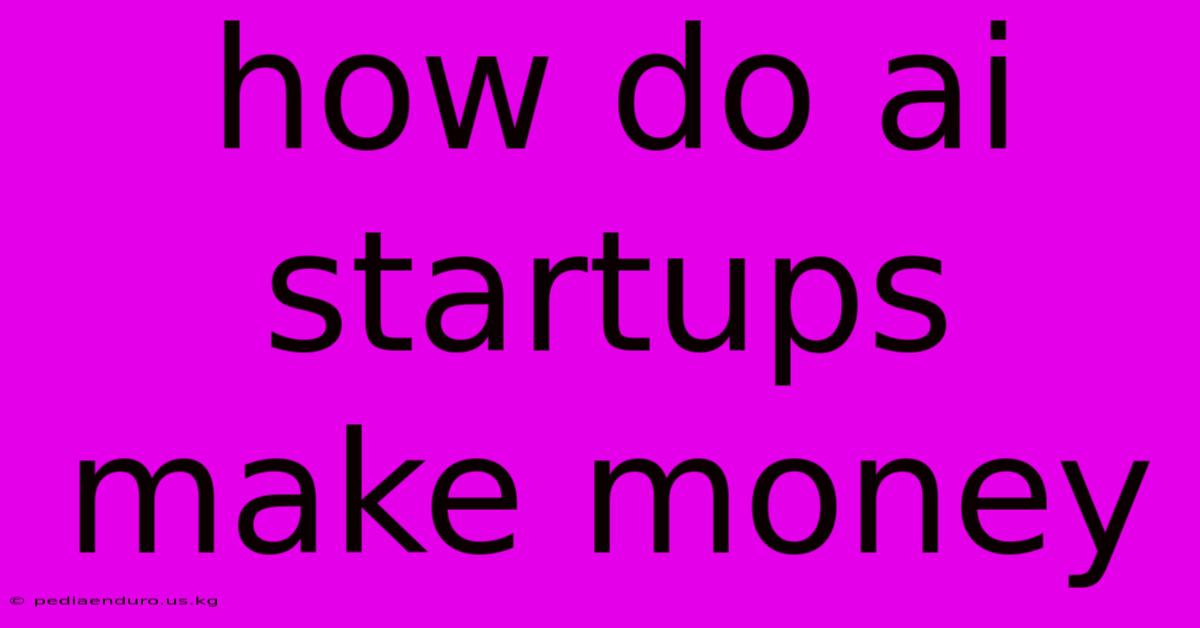
Thank you for visiting our website wich cover about How Do Ai Startups Make Money. We hope the information provided has been useful to you. Feel free to contact us if you have any questions or need further assistance. See you next time and dont miss to bookmark.
Also read the following articles
Article Title | Date |
---|---|
Censori Sa Grammy 2025 Isang Sorpresa | Feb 03, 2025 |
Nonton As Roma Vs Napoli Live Streaming | Feb 03, 2025 |
Liga Italia Derby Milan Seri Dramatis | Feb 03, 2025 |
Is There A Robot Emoji | Feb 03, 2025 |
Bing Ai Image Apk | Feb 03, 2025 |